The Impact of Artificial Intelligence on Business Analysis: A Brain-Friendly Adventure with Alex and James
- JACOB P JAMES
- Jun 29, 2024
- 5 min read
Meet Alex and James, two business analysts navigating the exciting world of data and technology. Alex, a seasoned professional with a touch of gray in his hair and wisdom in his eyes, loves sharing his knowledge. James, a bright-eyed newcomer, is eager to learn and explore new frontiers. Today, they’re diving into the fascinating topic of Artificial Intelligence (AI) and its impact on business analysis, especially for an IT business analyst working in an application team.

James: Hey Alex, I keep hearing about Artificial Intelligence. How is AI changing our jobs as business analysts, especially in an IT application team?
Alex: Great question, James! Think of AI as a superpower that’s transforming our toolkit. Imagine being able to analyze massive datasets in minutes or predict market trends with impressive accuracy. It’s like having a crystal ball and a super-speed detective all in one.
James: That sounds amazing! But how exactly is AI changing our day-to-day tasks, like collecting requirements or drafting BRDs?
Alex: Let’s break it down, James. First, there’s data analysis. AI algorithms can sift through mountains of data and find patterns faster than we ever could.
James: So, it’s like having a super-speed data detective on our team?
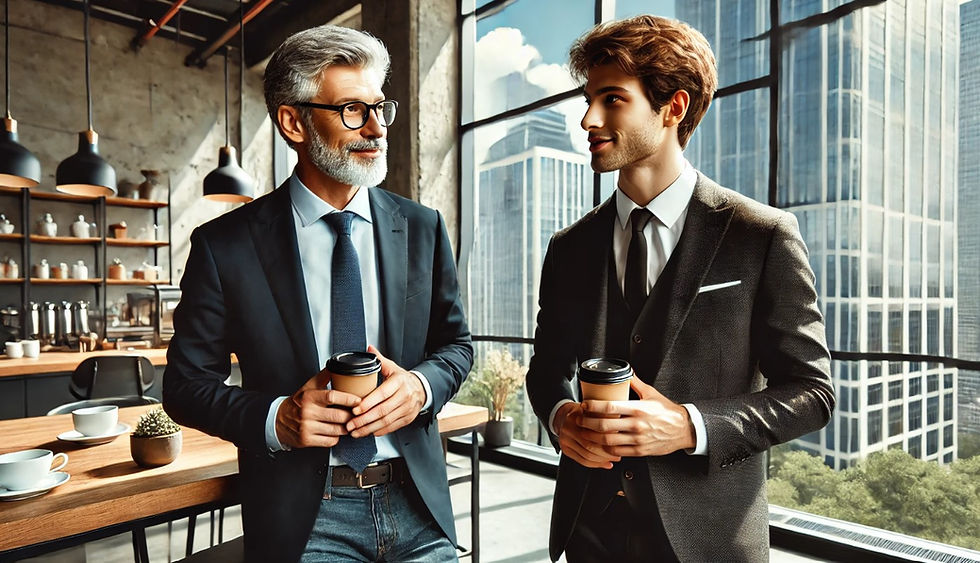
Alex: Exactly! When collecting requirements, AI can help by analyzing existing documentation, requirements, logs, and user feedback to identify common themes and pain points. This gives us a head start before we even begin our stakeholder meetings.
James: That would make drafting BRDs and user stories so much easier!
Alex: Definitely. Speaking of drafting, AI tools can assist in writing BRDs and user stories. Natural language processing (NLP) can help generate initial drafts by pulling in relevant data and structuring it coherently. It’s like having a smart assistant that helps you with the heavy lifting.
James: Talking about Natural Language Processing, or NLP. How does it actually work?
Alex: Great question, James! NLP is like giving computers the ability to understand, interpret, and respond to human language in a meaningful and useful way. Think of it as teaching a machine to understand and communicate like a human.
James: That sounds incredible! But how does it actually do that?
Alex: Let’s break it down. NLP involves several key steps: Tokenization, Parsing, Sentiment Analysis, and Entity Recognition.
James: James: Okay, let's take them one by one. What’s Tokenization?
Alex: Tokenization is the process of breaking down a text into smaller units called tokens, which can be words, phrases, or even entire sentences. For example, the sentence “AI is amazing” would be tokenized into “AI”, “is”, and “amazing”.
James: Got it. So, it’s like chopping text into bite-sized pieces?
Alex: Exactly. Next is Parsing, which involves analyzing the grammatical structure of a sentence. It helps the machine understand the relationships between words and their roles in a sentence.
James: So, it’s like diagramming sentences back in school?
Alex: Precisely! Then we have Sentiment Analysis, which determines the emotional tone behind a body of text. It’s used to identify opinions, emotions, and attitudes in the text.
James: That sounds useful for understanding customer feedback or social media posts.
Alex: Absolutely. Finally, there’s Entity Recognition, which identifies and categorizes key elements in the text, like names of people, companies, dates, and locations.
James: So, if a text mentions “Apple” and “Tim Cook”, NLP can recognize “Apple” as a company and “Tim Cook” as a person?
Alex: Exactly! And these components come together to help us make sense of large amounts of text data. For instance, tools like Google Cloud Natural Language API or IBM Watson can process and analyze text data, giving us valuable insights.
James: How can we use these insights in our work?
Alex: There are numerous applications. Let’s say we’re analyzing customer feedback. NLP can quickly process thousands of reviews, identifying common themes, sentiments, and key issues. This allows us to address customer concerns more effectively.
James: That would save us so much time! Any other examples?
Alex: Sure. In our role, we often draft BRDs and user stories. NLP can help by analyzing past documents and generating initial drafts. It can even suggest improvements or flag inconsistencies.
James: So, it’s like having an assistant that understands the language of business analysis?
Alex: Exactly! And don’t forget about chatbots. With NLP, we can create intelligent chatbots that understand and respond to user queries, providing support and gathering information around the clock.
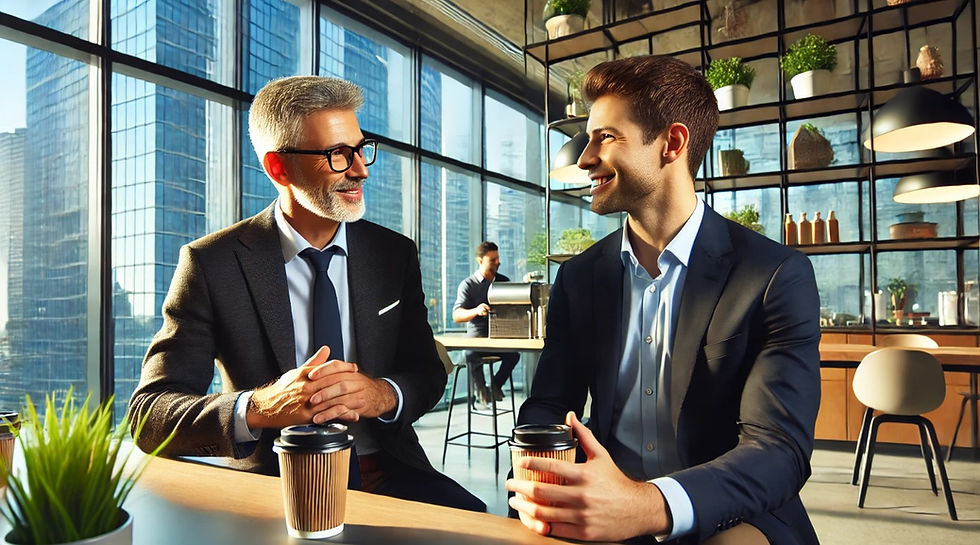
James: That sounds like a huge time saver. What about discussing requirements with developers?
Alex: Here’s where AI really shines. By using AI-driven analytics, you can provide developers with precise, data-backed requirements. AI can also help predict potential issues in development by analyzing historical data and identifying patterns that led to bugs or delays in the past.
James: So, it’s like having a crystal ball for development hiccups too?
Alex: Exactly! AI-driven analytics are incredibly powerful in our discussions with developers. Let's say we're working on a new application feature. AI can analyze historical data from previous projects and identify patterns that have caused issues before. For instance, if a specific type of integration has historically led to bugs, AI can flag this as a potential risk.
James: How does that work in practice?
Alex: Imagine we're developing a new payment processing feature. We can use an AI tool like Microsoft Azure AI to analyze past projects where we integrated payment gateways. If the AI finds that issues often occurred when integrating with certain types of third-party APIs, it can alert us. This allows us to discuss these potential trouble spots with the developers early on and adjust our development approach accordingly.
James: That sounds really helpful. Can you give another example?
Alex: Sure. Let’s say we’re adding a new customer feedback system. AI can look at previous feedback system implementations and notice that delays often happened during the user interface design phase. By using a tool like IBM Watson, we can analyse historical project data and predict these delays. Knowing this, we can preemptively allocate more resources or time to this part of the project, preventing delays before they happen.
James: So, it’s all about being proactive rather than reactive?
Alex: Exactly. This proactive approach is a game-changer. By using AI to anticipate and mitigate issues, we can keep projects on track and avoid the last-minute scrambles that often plague development cycles. Plus, developers appreciate having clear, data-backed requirements and early warnings about potential pitfalls. It makes their job easier and more predictable.
James: That would definitely streamline our workflow. But how do we get developers on board with using AI?
Alex: Good question. The key is to demonstrate the value. Show them how AI-driven insights can reduce their workload and improve the quality of the final product. Once they see the benefits in action, they’ll be more likely to embrace it.
James: This all sounds fantastic, Alex. But what about the human touch? Will AI replace us?
Alex: Not at all. AI is a tool to enhance our capabilities, not replace us. Our role as business analysts is still crucial because we bring context, empathy, and critical thinking to the table. AI can handle the heavy lifting, but we interpret the results and make strategic decisions.
James: That’s reassuring. So, in a way, AI is our trusty sidekick.
Alex: Exactly. Think of AI as Robin to our Batman. Together, we can achieve incredible things.
James: I’m excited to dive deeper into AI and see how it can boost our work. Thanks for the insights, Alex!
Alex: Anytime, James. Embrace AI, keep learning, and remember – with the right tools and mindset, there’s no limit to what we can achieve.
Comments